Generative AI: a problematic illustration of the intersections of racialized gender, race, ethnicity
NB: this post is a draft and subject to change; it forms a pre-print (an author’s original manuscript) I have authored.
Learning, teaching and technology have often been a big part of my career - since way back to the mid/late-2000s! Now in 2023, talk of artificial intelligence and education is omnipresent, and it's here to stay. Machine learning allows AI tools to become more intelligent by drawing on datasets to develop expertise over time. However, AI tools rely upon raw data created by humans; these datasets, in turn, reflect the biases of those who have gathered the evidence, which will be racial, economic and gendered in nature (Benjamin, 2019, p. 59).
Several researchers (Noble, 2018; Benjamin, 2019; Mohamed et al., 2020; Zembylas, 2023) are looking into the underpinning reasons that enable AI to skew results and create representations that overlook and erase others while focusing on specific, dominant groups. Specifically, the way that the human-created algorithms informing AI and generative AI tools portray racialized, gendered people is especially problematic. To understand why problematic representations of people are created, it is worth looking at the ideas of intersectionality (Crenshaw, 1991; hooks, b, 2015; Hill Collins, 2019). I draw on bell hooks and Patricia Hill Collins’s works here and recommend the reader acquaint themselves with Kimberlé Crenshaw’s work.
I write this post from my position as a part-time doctoral student, educator and higher education worker at a Scottish university in the UK. I write it as someone who’s interested in and curious about technology and as someone who teaches, develops, coaches. mentors educators (lecturers) how to teach and augment their teaching practices. However, I also write it from the perspective of a US migrant and dual national who has lived/worked in China, Russia, Kazakhstan and the UK. I note these as they inform my positionality when writing this post as I am interested in the interplay of education, culture, media representation, critical pedagogy and decolonial thinking as some of the ideas underpinning these areas inform some of my personal and professional values.
As a colleague of mine wrote "As per the Russell Group principles, I strongly believe it’s my job as an individual educator and our job as a sector to guide students how to use AI appropriately." I take their words and apply them to my own context: I believe it is my job as an educator to guide students and university staff in understanding and using AI appropriately.
For educators, this will give you an insight into some of the affordances of generative AI tools for creating images while exposing you to some of the opportunities and serious problems of using, for example, DALL-E, to create images. This post should give you ideas for developing your own practice with your students and your colleagues, no matter their experience as educators.
Thank you to colleagues and friends who have helped expand my thinking when writing this post.
Introduction
Using generative artificial intelligence (AI) tools can be exciting, confounding, scary and confusing. This was my experience and observation upon showing an academic colleague how a generative AI tool like ChatGPT can work by taking text prompts that are then create text-based content. Although text content creation tools have been at the forefront of everyone's mind since at least mid-2022, there are other generative AI tools that exist and merit attention. At the time of this writing, I can see common generative AI tools being categorized into three or four major types:
text to text (e.g. ChatGPT, Google Bard, Cohere.ai)
text to image (e.g. DALL-E, Midjourney, Stable Diffusion)
text to media, such as audio or video
and text-to-code, for coding and programming purposes
In this post, I focus on text-to-image generative AI through example prompts that I created. I analyze what it produced to demonstrate that educators must experiment with generative AI tools to understand and critique the tools and what they produce. In doing so, we can begin to understand how and why such tools create the content that they do. I use intersectionality as a heuristic (Hill Collins, 2019) to analyze the AI-generated avatars by looking at how these represent socially constructed identities in terms of racialized gender, race, ethnicity and nationality. Humans create algorithms and algorithms, in turn, create representations based upon human-created algorithms.
Specifically, we can deepen our understanding the reasons that generative AI tools (and other technologies) create questionable content that might, at the very least, underpinned by stereotypes representing an intersection of racism, misogyny, classism and/or xenophobia.
Finally, we must recognize that, for the moment, there is no concrete solution that a lay academic or layperson can implement to achieve this without a collective, concerted effort that includes a range of groups focused on shining light on the issues, changing hearts, minds and code and imaging ways forward to an equitable, inclusive world. Decolonial thinking can offer some imaginations to counter the coloniality of AI.
I first provide the context by laying out four (4) example prompts that I created an entered into DALL-E. I briefly touch on the prompts I created before moving on to analyze the results of each of the prompts. I provide a basic critique of the subsequently created representations by looking at the atmosphere, decor, clothing, facial expressions, ethnicity, or race.
For clarity, I use definitions of race and ethnicity offered by Fitzgerald (2020, p. 12) that sees race as referring to a ‘group of people that share some socially defined characteristics, for instance, skin color, hair texture, or facial features’ while ethnicity encompasses the ‘culture, nationality, ancestry and/or language’ shared by a group of people irrespective of their physical appearance (ibid). Grosfoguel offers another take on race informed by decolonial thinking: race is what he terms ‘a dividing line that cuts across multiple power relations such as class, sexual and gender at a global scale (2016, p. 11). In this case, race and subsequent racism are institutional and structural in nature in that the concept of race creates hierarchies of power and domination which are compounded by gender, sex, class and other factors.
While the concepts of race and ethnicity are social constructs and neither are mutually exclusive, I use these definitions to frame my analysis.
I highlight what is represented, and why the representations might appear this way and leave you, the reader, with critical questions to consider as you and your prospective students/learners explore the usage of generative AI for creating images from text. I then offer some possible solutions drawing on decolonial thinking.
NB: some readers will find the results disturbing, upsetting and potentially angering.
Sweet old grannies
Generative AI allows us to experiment with ideas to then create representations of those ideas, whether these are text, images or other media. In these short cases, I asked DALL-E to create illustrations of sweet old grannies making pancakes. As a reminder, DALL-E is one of three major text-to-image generative AI tools, and there are many others out there.
This was an impromptu idea that came up for a few reasons. In my current role, there is much discussion on the issues of generative AI and how to prepare students and educators. I also like pancakes and I have some fond memories of one of my grandmothers who would visit regularly when I was younger. I also worked and lived in Russia for a while where both pancakes and grandmothers are a big part of the culture. Pancakes are big around Maslenitsa or Carnival as it is known in other countries that celebrate the Western Christian version of the event, while grandmothers are a major cultural symbol, source of unpaid family work (Utrata, 2008) and symbol of stoicism that represents an intersection of age, gender and class (Shadrina, 2022). I also thought it would be playful and also allow me to see how DALL-E, a tool created by humans who programmed algorithms, would represent humans.
For transparency, I acknowledge that I am using gendered, ageist and even stereotypical language, especially in terms of describing 'a sweet, old X grandmother'. I am also aware that I am focused on a particular type of social/familial role, a grandmother. Not all old(er) women are grandmothers and not all grandmothers are old! As Benjamin (2019, pp. 102 drawing on Blay, 2011) asserts, qualifying words - those adjectival words used to describe 'opinion, size, age, shape, colour, origin, material, purpose' (Dowling, 2016) often encode gender; race, racism and racialization; and the humanity of individuals and groups of individuals (see Wynter, 2003).
Initial prompts
I used a prompt and only changed the adjectival qualifier describing the national origin of the imaginary character or avatar: "Create an image of a sweet, old X grandmother making pancakes". I tried out these prompts over a period of two weeks in July 2023. The queries I created are these:
"Create an image of a sweet, old Polish grandmother making pancakes"
"Create an image of a sweet, old Russian grandmother making pancakes"
"Create an image of a sweet, old American grandmother making pancakes"
"Create an image of a sweet, old Black American grandmother making pancakes"
I use specific terms to get the generative AI tool DALL-E to generate specific results to allow me to see what the AI tool produces so that I can then analyze the results. This, in turn, offers evidence and clues to understanding how human-created algorithms create the outputs that they do within generative AI tools.
In each case, DALL-E created four (4) individual representations of each character or avatar to illustrate the prompt I had created; in total, there are 16 images which you can see below with a caveat. Generative AI does not currently do well with the finer details of humans such as facial expressions, eyes, and hands. While I won't focus on hands and eyes specifically, facial expressions and ethnicity will be important later.
Representations of Polish and Russian grandmothers
At first glance, to the untrained eye and perhaps even to the untravelled eye, we might think nothing is amiss. There are four different images created that seemingly portray what is meant to be a sweet old Polish grandmother who is making pancakes, and another four representing Russian counterparts. Generative AI does not currently do well with the finer details of humans such as facial expressions, eyes, and hands. While I won't focus on hands and eyes specifically, facial expressions will be important later.
Atmosphere, decor, clothing
As we can see, each image illustrates a sweet, old Polish grandmother who appears to be in an almost gloomy environment. The lighting isn't bright but rather dark and almost shadowy. The representations of their Russian counterparts are very similar in many ways: the atmosphere is dark, perhaps gloomy. We can see what looks like wooden utensils being used and in some of the windows, we can see stereotypical lattice-type window net curtains.
Such portrayals could indicate a lack of modern lighting and/or electricity. The light also indicates the time of day, which could be an early morning golden hour, when they might rise to make an early morning breakfast. This does offer a stereotyped, ageist view of the women represented, however, by generalizing that all might rise at a very early hour to make pancakes.
If we look at the clothing, we see that each avatar is wearing clothing that is stereotypical of elderly Polish and Russian women: patterns that are floral in nature while headscarves. Some women do occasionally wear headscarves when attending church. However, these women are depicted in the home. However, we don't really get any indication of their hair or hairstyles, or whether these are things they might worry about simply because the representations cover or hide this particular aspect of all of these women.
In each case, it seems that perhaps these avatar-grandmothers are living in a different time based on the depictions of the atmosphere and technologies they are using. This doesn't mean that some do not live this way, however, it is problematic as certainly not all might live this way depending upon their means, wealth and family ties.
Expressions and ethnicity
The expressions of the Polish and Russian grandmothers are problematic for a few reasons. If we look at each of the women, most of them appear to be looking either down or away with only one of each looking ahead at the imaginary camera. The images as a collective might be seen to represent a sort of melancholic and depressing environment.
The women are either expressionless or perhaps seemingly unhappy in the eyes of someone from the US or UK apart from one of the Polish avatars. While there may be socio-historic rationales for portraying the women in such a way (e.g., World War I and World War II, followed by the Cold War) these images are explicitly problematic as they represent stereotyped, gendered and xenophobic representations of elderly Polish and Russian women.
In terms of ethnicity, for both the images representing these groups, all of the women are White or appear to be White. Poland, according to some statistics is 98% Polish so perhaps the representations are close to portraying the norm. On the other hand, Russia is more complex with its 193 ethnic groups yet the images portray a high level of homogeneity.
Ethnic Russians make up 77-81% of Russia's population of 147 million, along with Tatars, Ukrainians, Baskhirs, Chuvashs, Chechens and Armenians being other major ethnic groups of over a million (see https://en.wikipedia.org/wiki/Demographics_of_Russia#Ethnic_groups and https://minorityrights.org/country/russian-federation/ for a breakdown; there are other Russian-language sites that you can check as well). My point is here that Russia is a diverse nation of peoples of ethnic backgrounds and mixes including those of Slavic, Turkic, Caucasian, Mongolian peoples, indigenous and Korean ancestry. However, the images created by DALL-E portray avatars that represent only those who appear Slavic and/or European (i.e. White). There are no representations of other types of Russians who may be Turkic, indigenous, or Mongolian in origin.
However, this could be due to how algorithms encode the concept of a Russian person. Does 'Russian' mean a citizen of Russia, and therefore anyone who lives in Russia? If this is the case, then it is likely dominant views that inform datasets will skew any possible representations. On the other hand, does it mean those that see themselves as ethnically Russian? If this is the case, then perhaps it is valid to show only Slavic/European avatars. In either case, the representations are problematic as they highlight whatever the dominant 'norm' is while erasing Russia's historically rich diversity. Another perspective could be how a particular government might influence how the imaginations of its populace are portrayed, which may mean the prominence of a dominant group at the expense of an ethnic minority group. In Russia’s case, there are concerns surrounding ethnic separatism and how migrants are portrayed, especially of those from regions traditionally associated with Turkic and Asiatic peoples and those whose faith is Islam (Coalson, 2023). However, such concerns are not reasons for erasing different representations and portrayals of peoples of different ethnicities.
Representations of grandmothers from the US
As a reminder, I used the following prompt: "Create an image of a sweet, old American grandmother making pancakes". I acknowledge that using ‘American’ can be problematic. It can refer to people of the United States, or if you live in Latin America, American can refer to anyone from the Americas, not just people who live in the United States of America.
In addition to the term ‘American’ being problematic, this prompt quickly revealed more serious issues that I will touch upon.
Atmosphere, decor, clothing
The images of American grandmothers offer a stark contrast in many ways when compared with the representation of Polish and Russian grandmothers. The DALL-E produced illustrations appear to show these women, for the most part, in a different light.
While the first two women in the top row appear to be in the home, their homes appear to be more modern in some respects. They all appear to be using what appear to be metallic utensils as opposed to wooden ones. The lighting in the bottom two images is much brighter with almost an appearance of a representation of a cooking show as indicated by the lighter-colored walls. The atmosphere appears a lot less cluttered and lighter in many respects. This lack of clutter and more light might indicate, at the very least, modern homes that are efficient.
Then there are the hairstyles. These are, admittedly, something that I hadn't picked up on as it wasn't something that I am fully literate about until a friend prompted me. As that friend noted, the hair of these women tells another side of the story related to class. What does the hair say to you? How do each of their hairstyles represent their own lives? What does each style say about their socio-economic background?
The clothing also offers clues to how these avatar-representations live. Their clothes appear more modern, perhaps more expensive than their Polish and Russian counterparts. What does this say about the data that has informed the creation of these avatars?
Expressions and race
If we look at the facial expressions, again while generative AI does not yet get the finer details right, something appears and feels more warm, perhaps more positive about the expressions of these avatar women. The first one appears thoughtful and focused on what she is doing with almost a sense of enjoyment. The second one appears content - at the very least - with what she is doing. The third and fourth images appear to represent a wholly positive image of two different women engaging in cooking as indicated by slight smiles whether looking down (image 3) or looking straight ahead (image 4).
However, there is a significant problem with these representations which is indicated by the perceived race of the sweet, old, American grandmothers: each avatar represents a White woman. This is particularly problematic as the US has a population of over 330 million with nearly 80 million (nearly 1 in 4 people) who comprise non-White people. The question here then is why has the generative AI tool created only White faces to represent the qualifier ‘American’ when 1 in 4 people in the US fall under the broad categories of Black, Asian, Indigenous and others? Why is the US portrayed as, at least according to these AI-generated images, representing only one part of its population?
Representations of grandmothers from the US racialized as Black
Atmosphere, decor, clothing
If we consider the representations generated by DALL-E below, we see deeply problematic underlying issues that represent an intersection of race, gender and class in the portrayals of imagined sweet, old Black American women.
The atmosphere in each avatar appears generally warm and inviting, reflecting the representations of sweet, old, ‘American’ grandmothers. There is a certain simplicity and modernness to the environment. Two avatars appear in a home kitchen (the bottom two) as indicated by kitchen cabinets/cupboards and a nearby window. The top-left image appears perhaps in a larger, commercial kitchen or perhaps a kitchen in the home, and the second (top-right) appears perhaps in a TV studio as indicated by the lighting and focus.
One colleague, Dr Ruby Zelzer, notes something that I had missed:
… something struck me about how utilitarian the kitchens were, the kitchen tiles in 3 of the 4 pictures, and also that the type of tiles were very basic in appearance. How none of the other images had these tiles (to my eye).
However, the images appear to say something about the roles of these avatar women. Three of the images appear to represent the avatars as cooks or chefs, as indicated by what appear to be chefs' hats and their attire in general. The avatar in a pink apron and white outfit (top-right) appears to be in an ambiguous situation in part due to the lighting and the red nose: are they in a TV studio or in a circus? I will discuss this later as the representation harks back to minstrelsy and blackface.
In addition, two of the avatar women are wearing what look like cleaner gloves. The avatar portraying yellow gloves is also problematic as the gloves appear slightly worn and tattered. This can be seen to place someone, or here an older Black American woman, in a lower socio-economic position.
In 3 out of the 4 images (all bar the lower-left image), the avatars representing Black American grandmothers are situated in positions of service through the attire that they are portrayed to be wearing. In fact, only the avatar in a blue shirt and pink apron appears to be in a position that seemingly isn’t attributable to a service role. In contrast, the White representations of American women don’t appear to be in positions of service as indicated by their clothing. I now turn to discuss the problems that nearly all of these images is (re)producing.
Expressions and race
All the women appear to be smiling or enjoying what they are doing. At first, this may seem like a good thing. However, the expressions of the top-left and bottom-right avatars are highly problematic for a few historic reasons rooted in racist, gendered and classist portrayals of Black American women. In addition, the larger bodies of three of the other avatars also reflect how Black American women have historically been portrayed within the United States and beyond. In contrast, the avatars representing White American women are constructed with what appear to be more delicate and smaller features, something that several researchers (Bowdre, 2006; Downing, 2007; Thompson Moore, 2021) argue has frequently been attributed to representations of White women.
The origins of stereotyped representations of Black American women lie, in part, in minstrelsy in the 1800s (Bowdre, 2006; Downing, 2007; Thompson Moore, 2021). In minstrel shows, White men portrayed Black Americans by blackening their faces using burnt cork while exaggerating other facial features, such as the lips, by using 'red or white paint' (Bowdre, 2006, p. 37). The avatars representing Black American women are illustrative of how Black women were constructed in minstrel shows through the caricature of the wench (Thompson Moore, 2021, p. 318). White men performed the wench character representing Black women through cross-dressing and drag performances (ibid). Other characters would go further by dressing in 'brighter, more flamboyant dress' and their faces would be further exaggerated by makeup, creating 'larger eyes and gaping mouths with huge lips' (ibid). As Bowdre (2006) asserts, minstrelsy has aided stereotypes around people racialized as Black and continues to inform media representations of Black American men and women in the present day.
Another representation is that of Black American women as a ‘mammy,’ or a good-natured, submissive and motherly figure who would provide care for White families. Taken together, an excerpt from King (2019, p. 13) explains why such representations are deeply problematic:
“Aunt Jemima,” a well-known trope that (mis)represents/distorts Black/African womanhood in the USA, is a fictional historic advertising icon that reinforces the national stereotype of the slave plantation “mammy.” In the late 19th century, this image of a smiling, usually corpulent dark-skinned Black woman wearing a red bandana became the trademark logo for a technological innovation: ready-mixed pancake flour. Commercial advertisements that invented this denigrating image of Black womanhood expressed the white imagination, which was then reified in film, fiction, the fantasy world of plantation mythology, and consumer consciousness. This stereotype epitomises the dominance of hegemonic white memory and imagination in the material culture of American society (Wallace-Sanders 2008).
The images below depict what hooks (2015, pp. 65-66) would argue that such images portray Black women in a negative light through the construction of Black women having ‘excessive make-up,’ ‘wearing wigs and clothes that give the appearance of being overweight’ while simultaneously representing large ‘maternal figures’. bell hooks's message here is that historical depictions of Black American women portray them as fat/obese, older, asexual and unkempt, homogenizing this group while mocking them through the ‘wench’ and/or ‘mammy’ stereotypes, which both (re)produce demeaning representations of Black American women.
Discussion
What we see here in each of the images represents what are what Benjamin describes as (2019, p. 59) ‘deeply ingrained cultural prejudices’ and ‘biases’ drawn from data that the generative AI tools use to create representations.
While the imaginary representation of Black American women was reified in media and consumer consciousness, we can see that this portrayal resurfaces in the digital realm within the context of generative AI. What we see here then is one manifestation of ‘algorithmic coloniality’ (Mohamed et al., 2020; Zembylas, 2023). For those new to the concept of coloniality, this is a state of knowing and being that pervades knowledge and power relations that sees those formerly colonized and/or enslaved as regularly encountering inherent disadvantages in all aspects of life while former colonizers retain many advantages in all areas of life (Quijano and Ennis, 2000; Wynter, 2003; Ndlovu-Gatsheni, 2015). In simple terms, this means that accepted knowledges and ways of being represent those of the dominant members of society.
In this case, the role of Silicon Valley, located in the United States, which is a hegemonic power and an extension of the former European colonial nations as one of her settler-colonies, is significant. This extends beyond the technological companies of Silicon Valley and elsewhere in the US to anywhere that readily accepts, uses and replicates their models. Those who follow the dominant modes of cultural, and technological production take part in the creation and perpetuation of algorithms which overvalue some humans (those racialized as White) while undervaluing and actively devaluing the humanity of other humans (those racialized as Black, Asian and others).
Considering the #BlackLivesMatter movement and the daily injustices that people racialized as Black in the US (and elsewhere, even the UK for example) experience, it is particularly problematic that human-authored algorithms informing generative AI reflect dominant systems of knowing and being. It is, however, a testament to the existence of coloniality within AI and AI algorithms which (re)produce gendered, racist and xenophobic representations of racialized and minoritized peoples.
Although there is some hope for everyone to influence the datasets that inform algorithms, which in turn might allow for some change, this will not be easy: collaboration will be key and conscientization of everyone on the issues will be as well to address and rectify the issues of problematic algorithms, which are just one tool in a greater system.
Some specific solutions can help by drawing on decolonial thinking that can develop and deepen the understanding of students and educators. This can start with understanding where sites of coloniality replicate harmful generative AI algorithms. Drawing on Mohamed et al. (2020, p. 8)this might include understanding and identifying such sites, which might include where and how algorithms are made and function, who is involved in beta-testing and testing generally, and what local and national policies can be developed. This also includes specifically developing algorithmic literacy as part of digital literacy initiatives (Zembylas, 2023)
Key questions for students/educators
Why do the avatars represent these particular groups in the way that they do?
What, if anything, do the representations get right?
What, if anything, do the illustrations get wrong?
How are the representations problematic?
Where representations are problematic...
What message does this send to someone without knowledge of the context?
What message does this create about the people/cultures/objects portrayed in the images?
What can you do to ensure generative AI creates, if it is possible, more accurate and equitable representations of peoples/cultures/objects?
References
Benjamin, R. (2019). Race After Technology: Abolitionist Tools for the New Jim Code. Polity Press.
Bowdre, K. M. (2006). Racial mythologies: African American female images and *representation from minstrelsy to the studio era. [Doctoral dissertation/thesis, University of Southern California].
Coalson, R. (2023). Russia’s 2021 Census Results Raise Red Flags Among Experts And Ethnic-Minority Activists – RadioFreeEurope/RadioLiberty. Retrieved 2023-07-24 from https://www.rferl.org/a/russia-census-ethnic-minorities-undercounted/32256506.html
Crenshaw, K. (1991). Mapping the Margins: Intersectionality, Identity Politics, and Violence against Women of Color. Stanford Law Review, 43, No. 6, 1241-1299. https://doi.org/10.2307/1229039
Dowling, T. (2016, Tuesday, 13 September). Order force: the old grammar rule we all obey without realising – The Guardian. Retrieved 2023-07-24 from https://www.theguardian.com/commentisfree/2016/sep/13/sentence-order-adjectives-rule-elements-of-eloquence-dictionary
Downing, C. (2007). “Interlocking oppressions of sisterhood: (re) presenting the black woman in nineteenth century blackface minstrelsy”. Senior Scholar Papers, Paper 539. https://digitalcommons.colby.edu/seniorscholars/539
Fitzgerald, K. J. (2020). Recognizing Race and Ethnicity: Power, Privilege and Inequality (Third ed.). Routledge.
Grosfoguel, R. (2016). What is Racism. Journal of World-Systems Research, 22(1), 9-15. https://doi.org/10.5195/jwsr.2016.609
Hill Collins, P. (2019). Intersectionality as Critical Social Theory. Duke University Press.
hooks, b. (2015). Ain’t I a Woman: Black Women and Feminism. Routledge.
King, J. E. (2019). Staying Human: Forty Years of Black Studies Practical-Critical Activity in the Spirit of (Aunt) Jemima. International Journal of African Renaissance Studies - Multi-, Inter- and Transdisciplinarity, 14(2), 9-31. https://doi.org/10.1080/18186874.2019.1690399
Mohamed, S., Png, M.-T., & Isaac, W. (2020). Decolonial AI: Decolonial Theory as Sociotechnical Foresight in Artificial Intelligence. Philosophy & Technology, 33(4), 659-684. https://doi.org/10.1007/s13347-020-00405-8
Ndlovu-Gatsheni, S. J. (2015). Decoloniality as the Future of Africa. History Compass, 13(10), 485-496. https://doi.org/10.1111/hic3.12264
Noble, S. U. (2018). Algorithms of Oppression: How Search Engines Reinforce Racism. New York University Press.
Quijano, A., & Ennis, M. (2000). Coloniality of Power, Eurocentrism, and Latin America. Nepantla: Views from South, 1(3), 533-580.
Shadrina, A. (2022). Enacting the babushka: older Russian women ‘doing’ age, gender and class by accepting the role of a stoic carer. Ageing and Society, 1-18. https://doi.org/10.1017/s0144686x2200037x
Thompson Moore, K. (2021). The Wench: Black Women in the Antebellum Minstrel Show and Popular Culture. The Journal of American Culture, 44(4), 318-335. https://doi.org/10.1111/jacc.13299
Utrata, J. (2008). Babushki as Surrogate Wives: How Single Mothers and Grandmothers Negotiate the Division of Labor in Russia. UC Berkeley: Berkeley Program in Soviet and Post-Soviet Studies. https://escholarship.org/uc/item/3b18d2p8
Wallace-Sanders, K. (2008). Mammy: A century of race, gender, and southern memory. University of Michigan Press.
Wynter, S. (2003). Unsettling the Coloniality of Being/Power/Truth/Freedom: Towards the Human, After Man, Its Overrepresentation—An Argument. CR: The New Centennial Review, 3(3), 257-337. https://doi.org/10.2307/41949874
Zembylas, M. (2023). A decolonial approach to AI in higher education teaching and learning: strategies for undoing the ethics of digital neocolonialism. Learning, Media and Technology, 48(1), 25-37. https://doi.org/10.1080/17439884.2021.2010094
Creating a student induction with ChatGPT: what it does and misses
ChatGPT seems to be all the rage at the moment, and has been so since at least November/December of 2022. Students and educators are simultaneously worried, excited and fearful of the possibilities that a tool like ChatGPT might create. The inspiration for this post comes from a lot of thinking since at least February and March of this year, coupled with a couple of insightful blog posts from a colleague Dr Emily Nordmann.
What is ChatGPT?
Photo by Tim Mossholder on Pexels.com
I won't labor the point as this has already been talked about in many places, many times over the last few months. In my own words, ChatGPT a tool that draws upon a large set of information that is out there on the web while constantly being trained by its users to finesse what it produces for its users. Depending on whether you use the free or paid version, this information goes back to either 2021 at the time of this writing, or up to now when using the paid version.
The knowledge that ChatGPT draws on is shaped by a mixture of key factors that you should understand:
those who created the ChatGPT AI model - i.e. the programmers
the openly available information that ChatGPT is drawing from
the users whose queries and questions are training ChatGPT, in essence, to become better at what it is doing
Specific knowledge frames outputs
Understanding both what knowledge and whose knowledge informs ChatGPT, and any other tool, is important. When we lift the lid on such tools, we deepen our own understanding of how they work on multiple levels. This then allows us to understand how tools like ChatGPT might (and probably will) create potential omissions, erasures and bias in the knowledge that such tools produce.
With this in mind, I encourage you to try and test out such tools while also asking yourself:
Who has created this tool?
Where are they from?
What are their motivations?
What knowledge is this tool drawing on?
Whose knowledge is this tool drawing on?
What knowledge is missing? And why is it missing?
An example in practice: creating a student induction
Photo by DLKR on Pexels.com
I chose the image above to represent the first prompt and example. What you ask of a large language model like ChatGPT will directly influence the example answer you receive. So, the more vague your question is, the more encompassing the response you will receive.
For a practical example, I decided to get ChatGPT to create a student induction. I choose this example for a few reasons:
to understand what the tool will create using my question prompts
to share with educators an example of a student induction created by ChatGPT
to determine what could be missing from the example that ChatGPT creates
Initial prompt and first output
Below is what ChatGPT created from the initial prompt.
Me: Can you help me to create an induction program for first year students at a university?
ChatGPT: Absolutely, I'd be happy to help you design an induction program for first year university students. Here's a basic outline you could consider:
1. Welcome and Introduction (Day 1)
Welcome Speech: The head of the university or a senior faculty member can begin by welcoming the new students.
Introduction to University: Give an overview of the university's history, ethos, academic structures, key personnel, campus culture, and student life.
2. University Overview (Day 1 - Day 2)
Academic Session: Detail about the course curriculum, academic calendar, grading system, internships, research opportunities, and expectations.
Campus Tour: This could be conducted by senior students. Show important locations like lecture halls, library, labs, student support services, sports facilities, canteens, etc.
Introduction to Faculty: Introduce the teaching and administrative staff. This could be done departmentally.
Workshop on the University's Digital Infrastructure: Train students on how to use the university's website, student portal, learning management system, library resources, etc.
3. Student Services and Support (Day 3)
Presentation from Student Services: Discuss available services like counselling, career guidance, health, accommodation, international student services, etc.
Safety Briefing: Include information about campus safety, emergency services, important contact numbers, and any apps or systems used to enhance student safety.
Presentation from Financial Aid: Discuss scholarships, grants, loans, work-study opportunities, and other financial assistance available.
Presentation from Student Clubs: Representatives from various clubs and societies can introduce their activities.
4. Skills Workshops (Day 4 - Day 5)
Study Skills: Sessions on time management, effective study techniques, research skills, and academic writing.
Soft Skills: Workshops on communication skills, presentation skills, leadership, teamwork, etc.
Personal Development: Sessions on mental health awareness, stress management, maintaining a balanced lifestyle, etc.
5. Social Activities (Throughout the Week)
Ice Breakers and Team Building Activities: To help students get to know each other.
Welcome Party: A casual social event.
Quiz Night: A fun trivia night with questions about the university and general knowledge.
City Tour: For universities in or near cities, a tour of important city landmarks could be helpful.
Meet and Greet with Seniors: Casual interaction sessions with second-year students or student mentors.
6. Reflection and Feedback (End of the Week)
Reflection Session: Allow students to share their experiences and feelings about the first week.
Feedback Collection: Collect feedback about the induction program to improve future iterations.
Please note that this is a general schedule and might need adjustments according to the specific needs of your university and student population. The key is to provide essential information, introduce students to support services, foster community-building, and address any initial worries or concerns students may have.
ChatGPT will often end examples with a little statement like this
Some reflections
As we can see, this is a very detailed student induction for a week-long induction. My question was not specific and therefore the example it produced was broad and encompassing. The example likely draws on a range of university inductions for Year 1 students entering university from across the Internet. However, as we can see, the example is based upon a university that probably has stable electricity, Internet access and good facilities. It is a department within a university that is probably concerned with its reputation and so it takes strides to ensure the students get the best experience (services) possible.
Getting (more) specific
Photo by Lukas on Pexels.com
I wanted to understand how ChatGPT might compress or summarize the previous request. The aim was to understand what might change as a result.
This is only an example and I do not recommend a single day student induction!
Me: Thank you, can you regenerate this but for a 1 day program?ChatGPT
ChatGPT: Certainly, condensing the induction program into one day will require some adjustment, but it's still achievable. Here's a simplified structure:
1. Morning Session: Welcome and University Overview (9:00am - 12:00pm)
Welcome Address: The head of the university or a senior faculty member can begin by welcoming the new students (9:00am - 9:30am).
University Overview: A brief overview of the university's history, ethos, academic structure, key personnel, and student life (9:30am - 10:00am).
Academic Session: Detail about the course curriculum, academic calendar, grading system, and expectations (10:00am - 10:30am).
Short Break (10:30am - 10:45am)
Quick Campus Tour: Highlight the most important locations such as lecture halls, libraries, and student support services. This could be a virtual tour, if needed (10:45am - 11:30am).
Introduction to Faculty: A quick introduction to key faculty members and administrative staff (11:30am - 12:00pm).
2. Afternoon Session: Student Services and Workshops (1:00pm - 4:00pm)
Lunch Break (12:00pm - 1:00pm)
Presentation from Student Services: Highlight the main services like counseling, health, accommodation, etc. (1:00pm - 1:30pm).
Workshop on University's Digital Infrastructure: Train students on how to use the university's website, student portal, learning management system, etc. (1:30pm - 2:00pm).
Short Break (2:00pm - 2:15pm)
Essential Skills Workshop: A condensed workshop on essential academic skills like time management, effective study techniques, and academic writing (2:15pm - 3:00pm).
Safety Briefing: Share information about campus safety, emergency services, and important contact numbers (3:00pm - 3:30pm).
Q&A Session: Allow students to ask any remaining questions they might have (3:30pm - 4:00pm).
3. Evening Session: Social Activity and Close (4:00pm - 6:00pm)
Social Mixer: A casual event where students can interact with each other and faculty members. This could include light refreshments, ice breakers, or team building activities (4:00pm - 5:30pm).
Closing Address: A brief closing note from a senior member of the university staff, encouraging students to make the most of their time at the university (5:30pm - 6:00pm).
Remember, the aim is to provide essential information, help students feel comfortable in their new environment, and foster a sense of community. Be sure to let students know where they can find more detailed information or support if needed.
ChatGPT's disclaimer
Reflections on the details
When I saw this example, I was surprised and left wondering. What surprised me was the time that ChatGPT allocated to such sessions. This would be a highly regimented and time poor induction!
While the overall plan isn't bad, we know from practice that inductions can take a lot longer and might have unexpected events that just happen. Students and staff can get lost along the way, people might cancel and rooms might suddenly be unavailable due to unforeseen circumstances.
Testing ChatGPT for basic biases
Photo by Marta Nogueira on Pexels.com
I went one step further by asking ChatGPT what might be some good dedicated social media platforms for fostering community among the students. The idea is that these could be spaces where students connect pre-arrival. However, there were some problems in the examples that ChatGPT produced.
Here are some of the examples it suggested:
Facebook
WhatsApp
Discord
Slack
Instagram
LinkedIn
Twitter
Microsoft Teams or Google Workspace
Reddit
Clubhouse
SnapChat
TikTok
Perhaps the biggest problem with these examples is that they are all very popular and widely used in the West and perhaps particularly in the Anglophone and European West. However, to what extent is this inclusive of those from other regions of the world?
I had to prompt ChatGPT to get it to consider using Telegram and WeChat. For context, Telegram is popular in eastern Europe and the Middle East while WeChat is particularly popular in China and other parts of Asia. The graphic below provides one picture of how some suggestions that ChatGPT are perhaps good ones while others are missing.
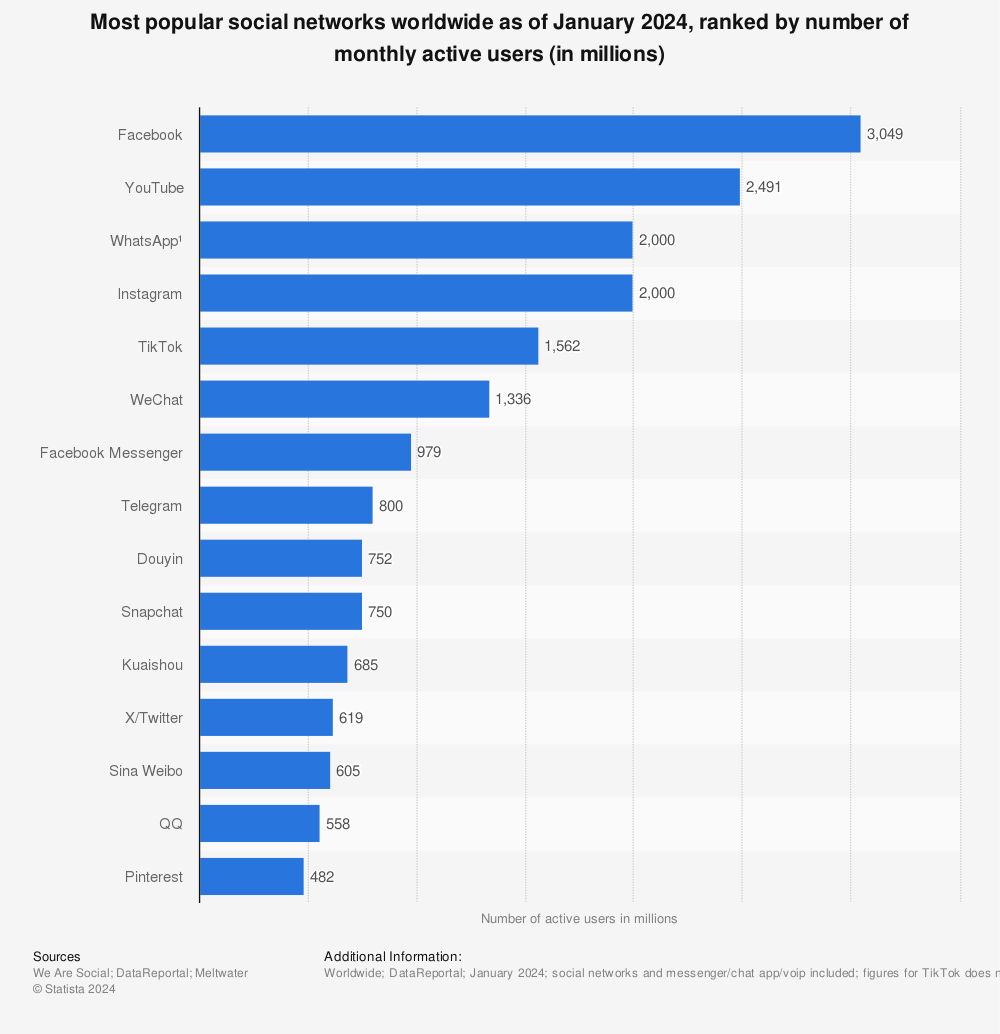
Find more statistics at Statista
Closing thoughts
What does this all mean for students and educators? I think it means that we need to do what we can and should be doing: when we use a tool, we should question critically who made it, what its purpose is and what it can and can't do. We should practice it, get to know it and see how well it works. Finally, we should question the information it is generating and be mindful of what is omitted and why it is missing.
For further information...
There are a lot of voices out there who are discussing ChatGPT and all of its potential. The two I have found most useful are those who have been consistent and have provided regular examples, while also creating space for dialogue.
One of the most consistent voices on the uses of ChatGPT on Twitter has been Mushtaq Bilaland one of the most regular organizers of voices has been Phil Anthony from the University of Kent who organizes regular Digitally Enhanced EducationWebinars.